

The variable time records survival time status indicates whether the patient’s death was observed ( status = 1) or that survival time was censored ( status = 0). The first thing to do is to use Surv() to build the standard survival object. Since ranger() uses standard Surv() survival objects, it’s an ideal tool for getting acquainted with survival analysis in this machine-learning age. Benchmarks indicate that ranger() is suitable for building time-to-event models with the large, high-dimensional data sets important to internet marketing applications. But ranger() also works with survival data. The ranger() function is well-known for being a fast implementation of the Random Forests algorithm for building ensembles of classification and regression trees. Ranger might be the surprise in my very short list of survival packages. Ggfortify enables producing handsome, one-line survival plots with ggplot2::autoplot. Thereafter, the package was incorporated directly into Splus, and subsequently into R. The first public release, in late 1989, used the Statlib service hosted by Carnegie Mellon University. Dr. Terry Therneau, the package author, began working on the survival package in 1986. Not only is the package itself rich in features, but the object created by the Surv() function, which contains failure time and censoring information, is the basic survival analysis data structure in R. The survival package is the cornerstone of the entire R survival analysis edifice. So, it is with newcomers in mind that I offer the following narrow trajectory through the task view that relies on just a few packages: survival, ggplot2, ggfortify, and ranger Newcomers - people either new to R or new to survival analysis or both - must find it overwhelming. As well-organized as it is, however, I imagine that even survival analysis experts need some time to find their way around this task view.
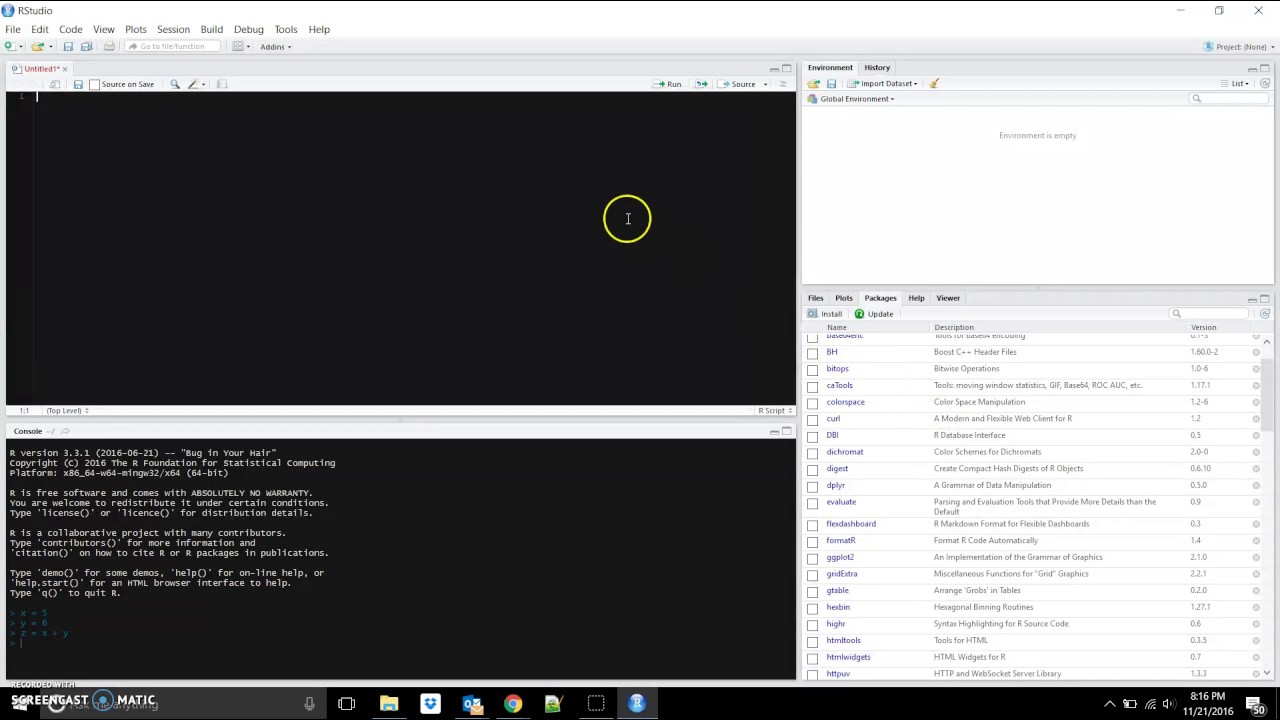
It is a fantastic edifice that gives some idea of the significant contributions R developers have made both to the theory and practice of Survival Analysis. Looking at the Task View on a small screen, however, is a bit like standing too close to a brick wall - left-right, up-down, bricks all around. We all owe a great deal of gratitude to Arthur Allignol and Aurielien Latouche, the task view maintainers. CRAN’s Survival Analysis Task View, a curated list of the best relevant R survival analysis packages and functions, is indeed formidable.
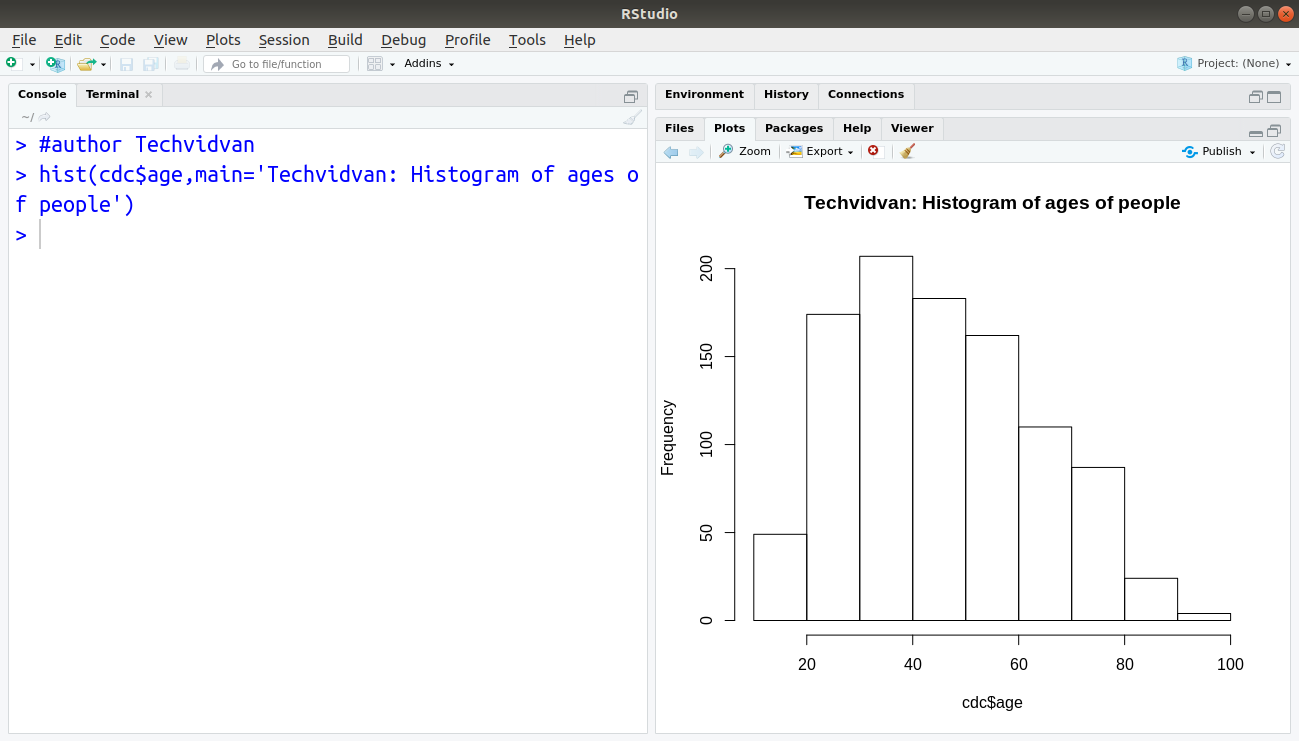
So, it is not surprising that R should be rich in survival analysis functions.
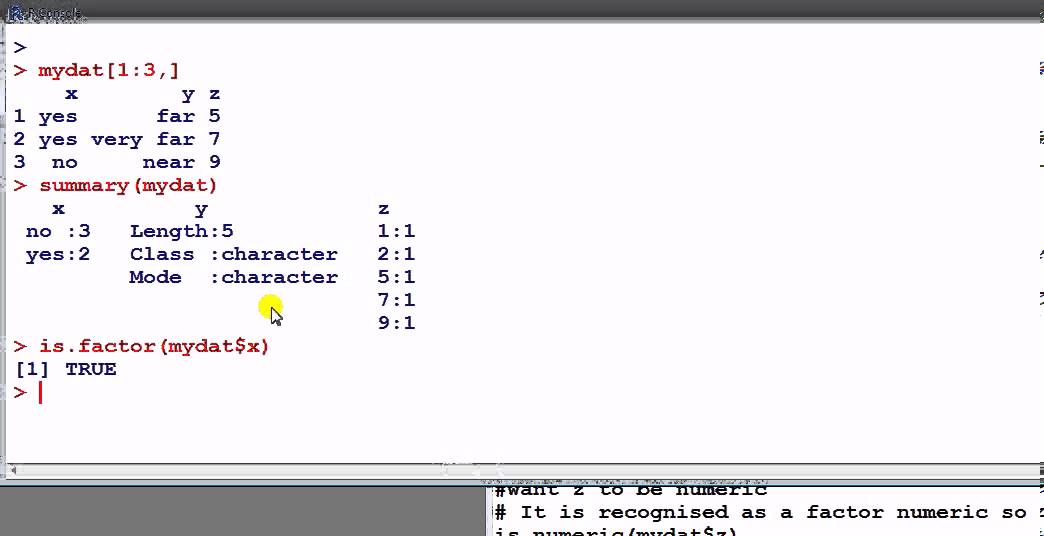
Today, survival analysis models are important in Engineering, Insurance, Marketing, Medicine, and many more application areas. Basic life-table methods, including techniques for dealing with censored data, were discovered before 1700, and in the early eighteenth century, the old masters - de Moivre working on annuities, and Daniel Bernoulli studying competing risks for the analysis of smallpox inoculation - developed the modern foundations of the field. With roots dating back to at least 1662 when John Graunt, a London merchant, published an extensive set of inferences based on mortality records, survival analysis is one of the oldest subfields of Statistics.
